Award for best paper to PhD student
PhD student Tiago Cortinhal from the School of Information Technology received the ”Best Student Paper Award” at the IJCAI 2021AI4AD workshop on Artificial Intelligence for Autonomous Driving.
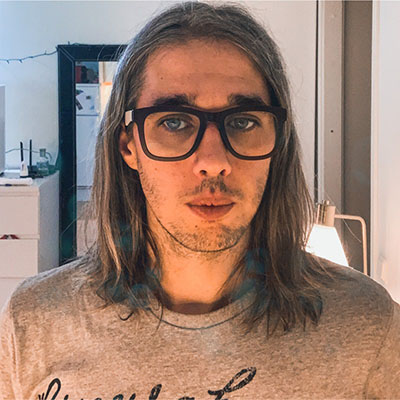
Tiago Cortinhal.
"Receiving this award was a happy surprise to me since it showed that this research is appreciated by my peers. This shows that the direction my work is taking is the right one. This recognition will fuel my motivation for the next steps, that's for sure!”, says Tiago Cortinhal, PhD student at the School of Information Technology and the Center for Applied Intelligent Systems Research, CAISR.
Tiago Cortinhal’s work is about semantics-aware multi-modal domain translation, that is generating 360-degree panoramic colour images from LiDAR point clouds. The paper is written together with Eren Erdal Aksoy, who is Tiago Cortinhal's PhD supervisor at Halmstad University, and Fatih Kurnaz at Middle East Technical University.
Tiago, tell us more about the reserach and results!
"The goal of any computer vision system employed in an autonomous vehicle is to ascertain the vehicle's surroundings. This is usually done by performing an abstraction over the data points each sensor is providing in an end-to-end approach by employing sensor fusion and Deep Learning Networks. In this scenario, and because of the sensor fusion, the segmentation network will be dependent on the availability of all sensor modalities, which could be problematic in the eventuality of a sensor failure.
In this paper, instead of recovering the failing modality directly, we exploit the more abstract semantic segments coming from the online sensor. This abstraction works by assigning a class to every single discrete point of data – in the case of an RGB image, each pixel will have a given category.
Having this abstraction as a middle step provides a "guiding line" to the learning task the network is facing, making the task itself easier than recovering the failing modality directly. It also allows us to have a less sensitive network to changes on the dataset because this abstraction is agnostic to those variations.
In the end, we show that the results when converting those semantic segments back to the sensor space looks more realistic than trying to recover the missing data directly. Still, we can also map the full 360º LiDAR scan to an RGB Image: effectively creating data, the network has never seen.
We have shown we can recover a missing modality by the intermediary of semantic segments. We can use our work to generate data that follows a different dataset distribution, which could be interesting from a data augmentation point of view."