Enabling the full potential of Machine Learning
In this era of big data, there is a need for intelligent systems that can improve continuously by learning from ubiquitous data streams. Mohamed-Rafik Bouguelia is developing Machine Learning algorithms that learn from large amounts of data and create useful knowledge – autonomously or with little human supervision. The research can for example be applied to predictive maintenance of complex systems, such as vehicles and district heating.
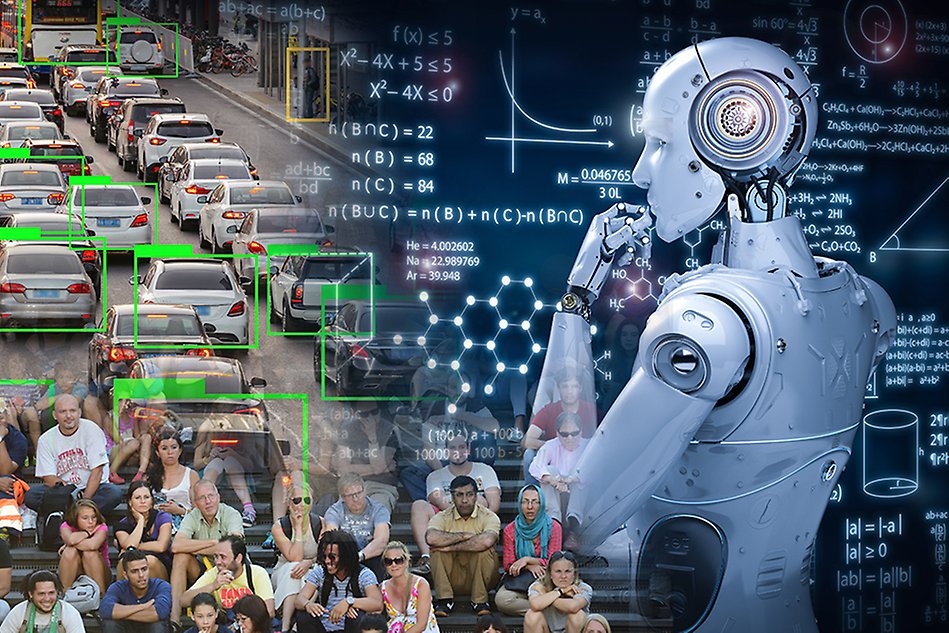
”Machine Learning is useful for a wide range of application domains. However, it still lacks the generalisability associated with human learning. The field would have a much broader impact if it could enable machines to easily interact with humans, adapt to changing contexts, and transfer knowledge between tasks.”
Mohamed-Rafik Bouguelia
When large amounts of data is collected and analysed through Machine Learning algorithms, useful information and knowledge can be generated. One example of such knowledge consists of detecting rare events and anomalies, for example deviations from any kind of normal behaviour or faulty equipment. Another example is the possibility to identify data clusters over different time scales and precision in multivariate time-series data. Such clusters can for example represent the operational behaviour of vehicles and machines, or human activities in a smart home. Extracting such high-level knowledge is essential to fully understand the operation of complex environments. But the techonology comes with several challenges, which constitute the core of the research conducted by Mohamed-Rafik Bouguelia, Associate Professor and Docent in Machine Learning at Halmstad University, Center for Applied Intelligent Systems Research (CAISR).
“Machine Learning is useful for a wide range of application domains. However, it still lacks the generalisability associated with human learning. The field would have a much broader impact if it could enable machines to easily interact with humans, adapt to changing contexts, and transfer knowledge between tasks”, says Mohamed-Rafik Bouguelia.
What is Machine Learning?
Machine Learning is a sub-field of artificial intelligence that gives machines the ability to learn and improve automatically through experience and by the use of data. Thus, instead of explicitly programming a machine to perform a task, it is programmed to learn how to perform the task. Machine learning algorithms build a model from ”training datasets”, then use this model to make predictions or take decisions.
Algorithms that adapt to change
The research of Mohamed-Rafik Bouguelia is conducted in collaboration with several Swedish industrial partners, for example Volvo Group, Alfa Laval and Öresundskraft. One of the research projects focuses on developing ’adaptive’ Machine Learning algorithms that can closely track and react to changes in the data distribution.
“Today, there are innumerous complex machines equipped with various sensors that collect data continuously over time. To detect anomalies or symptoms that indicate imminent failures, we design algorithms that can learn what constitutes a normal or abnormal behaviour from this data. However, such data streams are subject to change over time due to unforeseen external conditions. That is why we are working on algorithms that can adapt to data changes”, says Mohamed-Rafik Bouguelia.
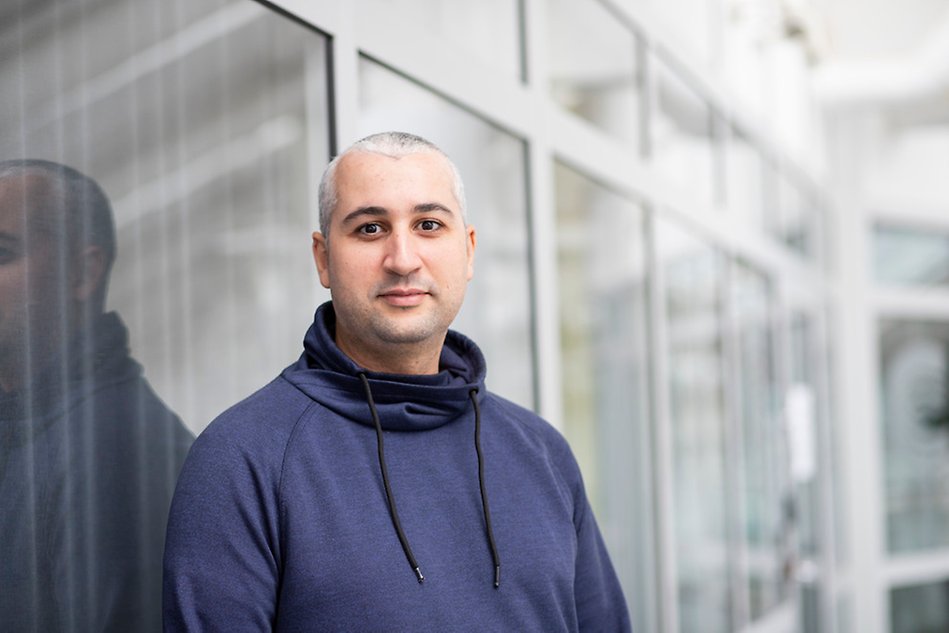
Mohamed-Rafik Bouguelia is an Associate Professor and Docent in Machine Learning at the School of Information Technology and the Center for Applied Intelligent Systems Research (CAISR) at Halmstad University.
About Mohamed-Rafik Bouguelia
- From Algiers, Algeria
- Born in 1987 in Nancy, France
- Education:
- Master's degree in Networks and Distributed Systems (2010) – USTHB University, Algeria
- Master's degree in Computer Science (2011) – University of Lorraine, France
- PhD in Computer Science (2015) – University of Lorraine, France (INRIA research center). Thesis topic: “Active learning from evolving data streams with uncertain expert knowledge”
- Started at Halmstad University in 2015 as a postdoctoral researcher
- Assistant Professor at Halmstad University until 2020
- Associate Professor and Docent in Machine Learning at Halmstad University from 2021
Human feedback is essential
Machine Learning algorithms can be used in many different areas, for example vehicles, trains, heat pumps and district heating. When monitoring district heating substations, for example, the data that is collected sometimes show atypical events that happen, but they are not necessarily anomalies. Anomaly detection is often subjective because what the user considers to be a ’relevant’ anomaly depends on the purpose of the application. Furthermore, real-world data may contain different plausible groupings, and a fully unsupervised clustering can not establish a grouping that suits the user’s needs because this requires external domain knowledge. Creating labelled data to train fully supervised machine learning algorithms is often costly and time-consuming, and such expert knowledge may not be available beforehand.
“To address these problems, we are developing interactive Machine Learning and Data Mining algorithms that include the human within the (machine) learning loop. For example, to distinguish between relevant and irrelevant anomalies, one can use algorithms that proactively communicate with a human expert to leverage her or his feedback and learn to suggest more relevant anomalies”, says Mohamed-Rafik Bouguelia.
The objective is not only to produce a more accurate anomaly detector, but also to minimise the expert effort required to investigate these anomalies. Other research directions along these lines include leveraging the human feedback to define most typical behaviours among a fleet of diverse systems, or to learn features and data representations that reveal faults and anomalies that are most relevant to the end-user.
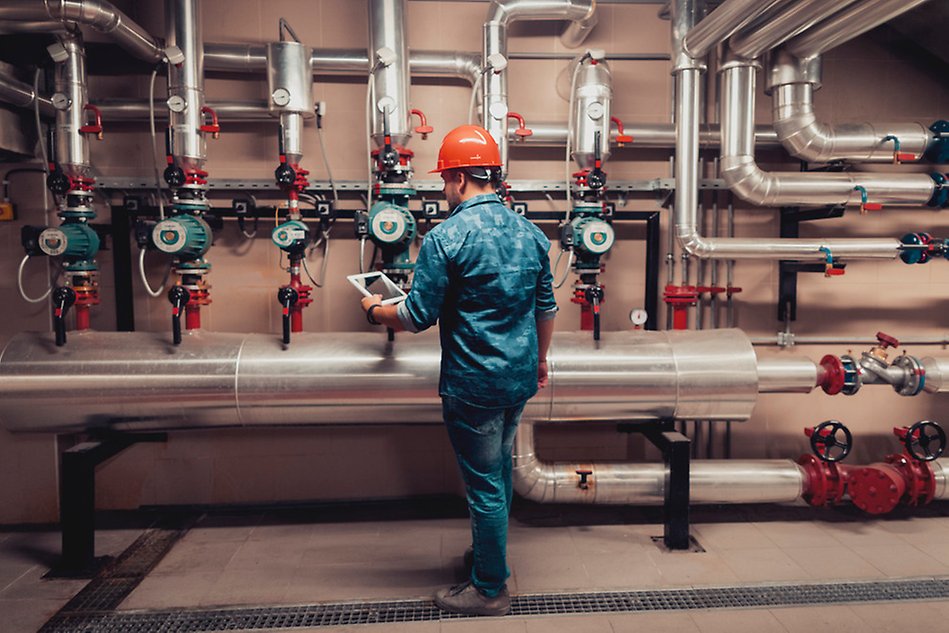
One application area for Machine Learning is within district heating. The algorithms can detect faults and failures that need to be controlled.
Multitask representation learning
Most existing Machine Learning algorithms assume that the data already has characterised features, designed manually by experts. This traditional way of preprocessing the data is not only tedious and time-consuming, but also not sufficient to capture all the different aspects of the available information. Moreover, the expert knowledge about the problem which allows them to come up with good representations does not necessarily generalise to other tasks. Currently, Mohamed-Rafik Bouguelia is focusing his research on designing algorithms for automatic learning of data representations that can be useful for multiple tasks.
“This is crucial for the continued success and broader use of Machine Learning algorithms as it allows for autonomous adaptation to a specific task by learning the most appropriate feature”, says Mohamed-Rafik Bouguelia.
This research direction is tightly connected to practical problems faced by several industrial partners that Mohamed-Rafik Bouguelia and his colleagues collaborate with. These industrial companies collect huge amounts of raw sensor data from for example heavy-duty vehicles, separators on marine vessels and smart buildings.
“This data needs to be mapped into a low-dimensional representation, as using the original raw data, for example for predictive maintenance, is not feasible. The overall goal is to automatically extract general features that are suitable for more than one task, for instance estimating the remaining useful life of several different components”, says Mohamed-Rafik Bouguelia and adds:
“All these research directions that we are working on can save both time and money, as well as contribute to a more sustainable industry.”
Text: Ragnhild Larsson and Louise Wandel
Photos: Ida Fridvall and Istock
Top illustration: Dan Bergmark